- Joined
- Apr 19, 2024
- Messages
- 179
- Reaction score
- 0
- Points
- 16
In today's fast-changing tech world, keeping AI apps safe is a top concern for developers and companies. AI is everywhere in our digital lives, making it crucial to protect these systems from harm. This guide will help you understand how to keep your AI apps safe, making them strong, reliable, and trustworthy.
Learn the basics of managing AI risks and how to model AI threats. Find out the best ways to secure AI app development. Also, explore the ethics of AI and the newest methods for checking for vulnerabilities. By the end of this guide, you'll know how to handle AI app security challenges. This will help you create a future where new ideas and safety go hand in hand.
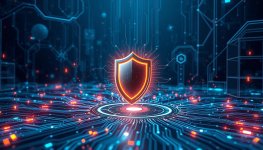
Understanding the Critical Need for AI Security in Modern Development
In today's fast-changing digital world, AI is becoming more common in apps. But, as we rely more on AI, we need strong security more than ever. AI security is not just important; it's essential for businesses and developers.Current Threats to AI Applications
AI apps face many security issues. For example, data poisoning attacks can harm an AI model's trustworthiness. Also, adversarial attacks can trick AI systems. These threats can damage the trust in AI solutions.Impact of Security Breaches in AI Systems
Security breaches in AI can harm businesses and users a lot. They can cause financial losses and damage a company's reputation. AI risk management and ai threat modeling are key to preventing these problems.Evolution of AI Security Challenges
As AI grows, so do the security challenges. AI vulnerability assessment must keep up to protect AI apps. This ensures they stay safe against new threats.Protecting AI apps is now a must in our digital world. By focusing on ai risk management, ai threat modeling, and ai vulnerability assessment, businesses can use AI safely. This way, they can benefit from AI while keeping their data and customers' trust safe.
Fundamental Principles of AI Risk Management
Protecting your AI applications is key. Understanding the core of AI risk management is the first step. It's important to focus on ai risk assessment, ai security best practices, and ai attack surface reduction.Start by finding and checking the risks in your AI systems. This includes looking at data integrity, model weaknesses, and AI system complexity. Knowing these threats helps you create strong defense plans for your AI apps.
It's also crucial to keep updating your risk management. The world of ai security best practices is always changing. You need to stay alert and adjust your ai risk assessment plans to keep up.
Reducing the attack surface of your AI systems is another key part. This makes your AI more secure against hackers and unauthorized access.
Using a complete AI risk management plan is essential. It covers identifying, assessing, mitigating, and monitoring risks. By following these principles, you can manage AI risks well. This keeps your users, data, and business safe.
Essential Components of AI Threat Modeling
Creating secure AI apps needs a strong threat modeling process. This step is key to spotting attack paths, setting up security measures, and ranking risks. Knowing the key parts of AI threat modeling helps you fix vulnerabilities and make your AI systems safer.Identifying Attack Vectors
The first thing in AI threat modeling is to carefully look at your AI app. You need to find out where hackers might target it. This includes checking data inputs, machine learning models, and how decisions are made. Understanding ai vulnerability testing and ai penetration testing helps you find and fix weak spots.Mapping Security Controls
After finding attack paths, you need to set up the right security controls. This might mean using secure ai coding practices, setting up advanced access controls, or using real-time monitoring. By matching your security with the threats your AI faces, you can protect it well.Risk Prioritization Strategies
After spotting attack paths and setting up security, you must rank risks. This means focusing on the biggest security threats first. A good threat modeling process helps make AI apps strong and safe against cyber attacks.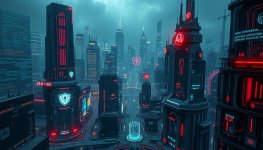
"Effective AI threat modeling is the foundation of secure AI development. By proactively addressing vulnerabilities and aligning security controls with identified threats, you can create AI applications that are resilient and trustworthy."
Securing AI App Development: Best Practices and Standards
Today, making ai application hardening secure is key. AI apps are everywhere, and keeping them safe is a must. You need to use strong secure coding practices from start to finish.Securing AI apps starts with checking user input. This stops attacks like SQL injection and XSS. Also, good error handling and access control reduce security risks.
Following ai ethics and governance rules is vital. This means privacy-by-design and responsible AI development. It builds trust and lowers the chance of bad outcomes.
Best Practices | Standards |
---|---|
|
|
Implementing Robust AI Vulnerability Assessment Protocols
Artificial intelligence (AI) is becoming more common, and keeping it safe is key. To protect your AI, you need strong vulnerability assessment protocols. This part will show you how to manage AI risks well.Automated Security Scanning
Tools for scanning AI systems are a great start. They use smart algorithms to find weaknesses in your AI code. By using these tools early, you can fix problems before they cause big issues.Manual Code Review Techniques
Manual code reviews add more depth to your AI security checks. Experts look closely at your AI code for any weaknesses. They check for data handling mistakes or logic errors that could harm your ai vulnerability management.Security Testing Frameworks
Using special security testing frameworks for secure machine learning helps a lot. Tools like AI-SEC and ARMOUR guide you in finding and fixing risks. They help you see where attacks might come from and how to stop them.Adding these protocols to your AI development process makes your apps safer. This keeps your users, data, and ideas safe from threats. Being proactive in managing vulnerabilities is essential for creating reliable AI solutions.
AI Data Privacy Protection Measures
In the world of AI, keeping data safe is key. AI systems gather and use lots of personal info. So, it's vital to have strong ai data privacy steps to keep users' trust and follow data rules.Data encryption is a big part of ai privacy protection. It makes sure data is safe, even if it gets stolen. Also, hiding personal info with techniques like data masking helps keep things private.
How data is stored is also important for ai ethics and privacy. This includes using strong passwords, making backups, and using secure cloud services. Following rules like GDPR and CCPA is also crucial for AI to be used right.
Putting privacy-by-design into AI making helps solve ai data privacy issues. This way, data stays safe from start to finish. It builds trust in AI technology.
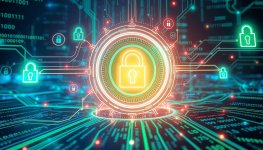
AI Privacy Protection Measure | Description |
---|---|
Data Encryption | Protecting sensitive data used in AI development and operations through encryption techniques. |
Anonymization | Removing or obscuring personally identifiable information from data to protect user privacy. |
Secure Data Storage | Implementing best practices for storing and managing AI-related data, including access controls and secure cloud solutions. |
Compliance with Regulations | Ensuring that AI development and deployment adhere to data protection regulations like GDPR and CCPA. |
Privacy-by-Design | Embedding privacy considerations into the entire AI development lifecycle to proactively address ai data privacy concerns. |
Ethical Considerations in Secure AI Development
As AI technology advances, we must think about its ethics. In secure AI development, we need to focus on privacy, ethics, and responsible use. These are key to making AI trustworthy and reliable.Privacy-by-Design Principles
Privacy-by-design is a must in AI development. It means adding data protection and user control from the start. This way, AI systems respect privacy and keep personal info safe.Ethical Guidelines Implementation
Setting up ethical guidelines is vital for secure AI. These guidelines should cover fairness, bias, and AI's impact on society. Following these, AI won't harm society or violate human rights.Responsible AI Development
Responsible ai ethical development means being open and accountable. It's about understanding how AI affects society. By doing this, AI systems gain trust from users and stakeholders.Putting ethics at the core of AI development is essential. By focusing on privacy, ethics, and responsibility, we create AI that's not just secure but also good for everyone.
Secure AI Model Deployment Strategies
Deploying AI models securely is key to protecting your AI applications. Start by using strong model versioning. This lets you track and manage different model versions easily. Also, store your trained AI models in safe places, controlling who can access them and watching for any changes.To protect your AI apps, set up secure inference endpoints. These endpoints check users and decide who can use your AI services. Always watch how your models perform and update them quickly if needed. This way, you keep your AI solutions safe and reliable over time.
Keeping your AI lifecycle secure is vital for trust in your AI products and services. Always be on the lookout, update your AI models and apps regularly. Follow the best practices for secure AI deployment to protect your business from threats.
FAQ
What are the current threats to AI applications?
Threats to AI include data poisoning and adversarial attacks. Data poisoning happens when bad actors mess with training data. This can make AI models act wrongly. Adversarial attacks trick AI systems into making bad predictions.What is the impact of security breaches in AI systems?
Breaches in AI systems can cause big problems. They can lead to data leaks and fines. They also hurt a company's reputation and trust from customers.Bad AI decisions can disrupt operations and even cause harm.
How can I implement effective AI risk management practices?
To manage AI risks, first identify and assess risks. Then, find ways to lessen their impact. This means making AI systems less vulnerable and using strong security measures.Keep an eye on risks and update your strategies as needed.